Introduction to Data Science
Data science has emerged as a pivotal discipline in today’s data-driven world, encompassing a variety of methodologies and techniques used to extract insights and knowledge from structured and unstructured data. At its core, data science integrates principles from statistics, computer science, and domain expertise, thus forming an interdisciplinary framework that addresses diverse challenges and phenomena across multiple industries.
The evolution of data science can be traced back to the early days of statistics, where the focus was primarily on information gathering and preliminary analysis. As technology advanced, particularly with the advent of big data and machine learning, the field expanded considerably. This transformation cultivated the emergence of specialized roles known as data scientists, whose responsibility lies in analyzing vast datasets using sophisticated algorithms and computational techniques to inform decision-making processes. Consequently, a confiler between analytical methods and real-world applications has been established, whereby organizations leverage data insights to enhance operational efficiency and foster innovation.
In recent years, data science has become increasingly significant, impacting various sectors such as finance, healthcare, marketing, and logistics. Organizations recognize the value of data-driven strategies, leading to an accelerated demand for skilled professionals who can navigate through complex datasets and derive actionable insights. As companies aim for more robust decision-making processes, the role of data science has evolved into a strategic asset, paving the way for competitive advantages.
Furthermore, as we continue to generate and accumulate massive volumes of data, the necessity for clear understanding and effective utilization of this information is paramount. The ability to harness data science is no longer optional; it is a critical component of how businesses navigate their operational landscapes, ultimately illustrating the essential nature of data science in modern decision-making frameworks.
Core Components of Data Science
Data science is a multi-disciplinary field that encompasses a variety of core components. Each element works synergistically to transform raw data into actionable insights, facilitating informed decision-making in various sectors. The primary areas of focus in data science are data collection, data cleaning, data analysis, and data visualization.
The first component, data collection, involves acquiring data from diverse sources. This could include databases, online surveys, or real-time data streams. The quality of insights derived from data science heavily relies on the adequacy and representativeness of the collected data. Therefore, robust data collection strategies are fundamental for any data-driven project.
Following data collection is data cleaning, a vital step that ensures accuracy and reliability in the dataset. Raw data is often riddled with inconsistencies, errors, and missing values. Through data cleaning processes, such as removing duplicates and correcting inaccuracies, data scientists prepare a clean dataset that forms the foundation for subsequent analysis. An effective data cleaning process enhances the overall productivity of data science efforts, as it directly impacts the results of the analyses conducted.
The next component, data analysis, involves applying statistical methods and algorithms to extract patterns and insights from the data. This phase may include exploratory analysis, predictive modeling, and machine learning techniques. The ability to conduct comprehensive data analysis enables organizations to forecast trends, identify correlations, and optimize processes, thereby driving more effective decision-making.
Lastly, data visualization is crucial for communicating the insights gained through data science. Effective data visualization employs graphical representations such as charts, graphs, and dashboards, making complex datasets more understandable. By presenting findings in a clear and visually appealing manner, stakeholders can grasp essential information quickly, facilitating informed decision-making.
In conclusion, the core components of data science—data collection, data cleaning, data analysis, and data visualization—are interdependent elements that work together. Understanding these components is essential for leveraging data science effectively in modern decision-making processes.
Data Science Methodologies
Data science encompasses a variety of methodologies that play a crucial role in deriving insights from complex data sets. Among the most significant methodologies are statistical analysis, machine learning, and predictive modeling. Each of these approaches offers unique capabilities and applications that contribute to the broader function of data science in modern decision-making.
Statistical analysis serves as the foundation of data science, providing a framework for interpreting data and making informed decisions. It involves collecting and analyzing quantitative data to uncover trends, relationships, and patterns. For instance, businesses often utilize statistical analysis to evaluate customer satisfaction through surveys, enabling them to understand consumer behavior and improve service delivery.
Machine learning, a subset of artificial intelligence, enables systems to learn from data and improve their performance over time without being explicitly programmed. This methodology employs algorithms to identify patterns and make predictions based on historical data. For example, in the finance sector, machine learning can be utilized to detect fraudulent transactions by analyzing unusual patterns in credit card usage, thereby enhancing security and reducing financial losses.
Predictive modeling is another important methodology within data science that utilizes statistical techniques to forecast future outcomes based on historical data. This can be invaluable for businesses in various industries, such as retail, where predictive models can project inventory needs and optimize supply chain management. By refining the decision-making process, organizations can reduce costs and increase operational efficiency.
In conclusion, the application of statistical analysis, machine learning, and predictive modeling illustrates how diverse methodologies in data science contribute to informed decision-making. By leveraging these approaches, organizations can harness the power of data to solve real-world problems and gain a competitive edge in their respective fields.
Role of Data Science in Business
Data science has emerged as a pivotal component in shaping modern business operations and decision-making processes. As organizations increasingly rely on data-driven strategies, understanding the role of data science becomes crucial for achieving operational efficiency and competitive advantage. By leveraging data, businesses can refine their marketing efforts, enhance customer segmentation, and optimize their resource allocation.
One of the primary applications of data science in business is in data-driven marketing. Companies collect extensive customer data through various channels, including social media, online transactions, and customer feedback. Data scientists analyze this information to identify trends and preferences, enabling businesses to tailor their marketing campaigns to target specific demographics more effectively. For instance, a retail company may utilize predictive analytics to anticipate customer behavior, allowing for personalized promotions that can significantly improve conversion rates.
In addition to marketing, data science plays a vital role in customer segmentation. By employing machine learning algorithms, businesses can cluster their customer base into distinct profiles based on purchasing behaviors, interests, and feedback. This segmentation allows companies to craft targeted marketing strategies and improve customer experiences. For example, an e-commerce platform might use clustering techniques to identify high-value customers and create loyalty programs designed specifically for them, thus fostering stronger relationships and increasing lifetime value.
Operational efficiency is another area where data science significantly impacts business. By analyzing performance metrics and operational workflows, companies can pinpoint inefficiencies and areas for improvement. Case studies have illustrated how organizations harness data science to streamline supply chain processes, reduce costs, and improve service delivery. Companies like Amazon have excelled in this domain by optimizing inventory management through predictive analytics, ensuring timely deliveries and customer satisfaction.
Through the effective harnessing of data, organizations across various sectors demonstrate that integrating data science into their operations leads to profound outcomes and a data-informed approach to decision-making.
Challenges Faced in Data Science
Data science has emerged as an integral component in modern decision-making, yet it is not without its challenges. One of the foremost issues is data privacy. With the ever-increasing volume of data being generated, there are substantial concerns regarding the protection of personal information. Organizations must navigate a complex landscape of regulations, such as the General Data Protection Regulation (GDPR), which mandates stringent data handling and processing guidelines. Consequently, data scientists must implement robust privacy measures to ensure compliance while still harnessing data for actionable insights.
Another significant challenge lies in data quality and accessibility. Often, the datasets used for analysis may contain errors, inconsistencies, or missing values, which can adversely affect the outcomes of data science projects. Advanced techniques such as data cleansing and transformation are essential to prepare the data adequately. Moreover, limited access to high-quality datasets can pose a hurdle, especially for smaller organizations or those restricted by budget constraints. This limitation can hinder the in-depth analysis necessary for informed decision-making.
Integrating data science into existing business practices also presents hurdles for many organizations. It requires a cultural shift within the company, fostering an environment that values data-driven decision-making. Often, there is resistance from stakeholders who may not fully understand the benefits of data science. To overcome this, it is crucial to educate employees across various departments about the value of using data effectively. Additionally, aligning data science initiatives with business objectives is paramount to ensure that analytics efforts genuinely contribute to organizational goals.
Addressing these challenges is crucial for professionals engaged in data science to maximize its potential. By embracing strategies that mitigate data privacy risks, enhance data quality, and facilitate integration, organizations can make more informed decisions and leverage the full power of data science.
The Future of Data Science
The future of data science is set to be shaped significantly by emerging technologies and trends that are currently transforming various industries. One of the most notable advancements is the rise of artificial intelligence (AI). AI technologies are enhancing data science capabilities, allowing for more sophisticated analysis and richer insights from vast datasets. As algorithms become increasingly adept at processing and interpreting data, their applications in fields such as healthcare, finance, and marketing are poised to expand dramatically.
Another critical component driving the future landscape of data science is the proliferation of big data analytics. With the exponential growth of data generated across different sectors, the demand for analytic tools that can efficiently handle and derive meaning from large volumes of information is increasing. Businesses are becoming more reliance on data-driven decision-making, necessitating the need for advanced analytics solutions that can facilitate real-time insights, predictive modeling, and enhanced performance metrics.
Moreover, automated machine learning (AutoML) is emerging as a pivotal innovation within the data science domain. This technology allows users, even those with limited technical expertise, to build and deploy predictive models without an extensive background in programming or statistical analysis. AutoML democratizes access to data science capabilities, thus fostering a broader implementation of data-driven strategies in organizations of varying sizes. As such, we can anticipate a future in which data science tools become more intuitive and user-friendly, magnifying the role of data in strategic decision-making.
The continued evolution within the data science field not only highlights the growing interconnection between technology and analytics but also suggests that organizations must adapt to these shifts. Adapting data science practices according to emerging trends will be crucial for operational efficiency and maintaining a competitive edge in a data-centric economy.
Real-World Applications of Data Science
Data science has proliferated across various sectors, shaping decision-making and transforming the way organizations operate. In healthcare, data science techniques are utilized for predicting patient outcomes, personalizing treatment plans, and managing hospital resources efficiently. For instance, machine learning algorithms analyze vast amounts of patient data to identify trends and predict the likelihood of diseases, enabling proactive measures and better patient care. This not only emphasizes improved health outcomes but also optimizes the allocation of medical resources.
Similarly, in the finance industry, data science plays a crucial role in risk assessment and fraud detection. Financial institutions utilize advanced analytics to evaluate credit scores, monitor transactions in real-time, and identify suspicious activities. By harnessing the power of predictive modeling, banks can enhance their strategies for loan approvals, ensuring that they are extending credit to reliable borrowers while minimizing losses from defaults. Additionally, investment firms rely on data science to analyze market trends and inform trading strategies, thereby gaining a competitive edge in a rapidly changing environment.
The retail sector also benefits substantially from data science applications. Retailers employ data analysis to enhance customer experiences through personalization. By examining purchasing behaviors and preferences, companies can tailor marketing strategies and offers to individual customers. Moreover, data analytics aids inventory management by predicting demand patterns, ensuring that products are available without overstocking. Through such practices, businesses can streamline operations and foster customer loyalty in a highly competitive marketplace.
Overall, the integration of data science in various industries demonstrates its capability to address complex challenges, enhance operational efficiency, and foster improved customer interactions. These real-world applications symbolize the significance of data science as a decision-making powerhouse, resonating with the evolving demands of the modern economy.
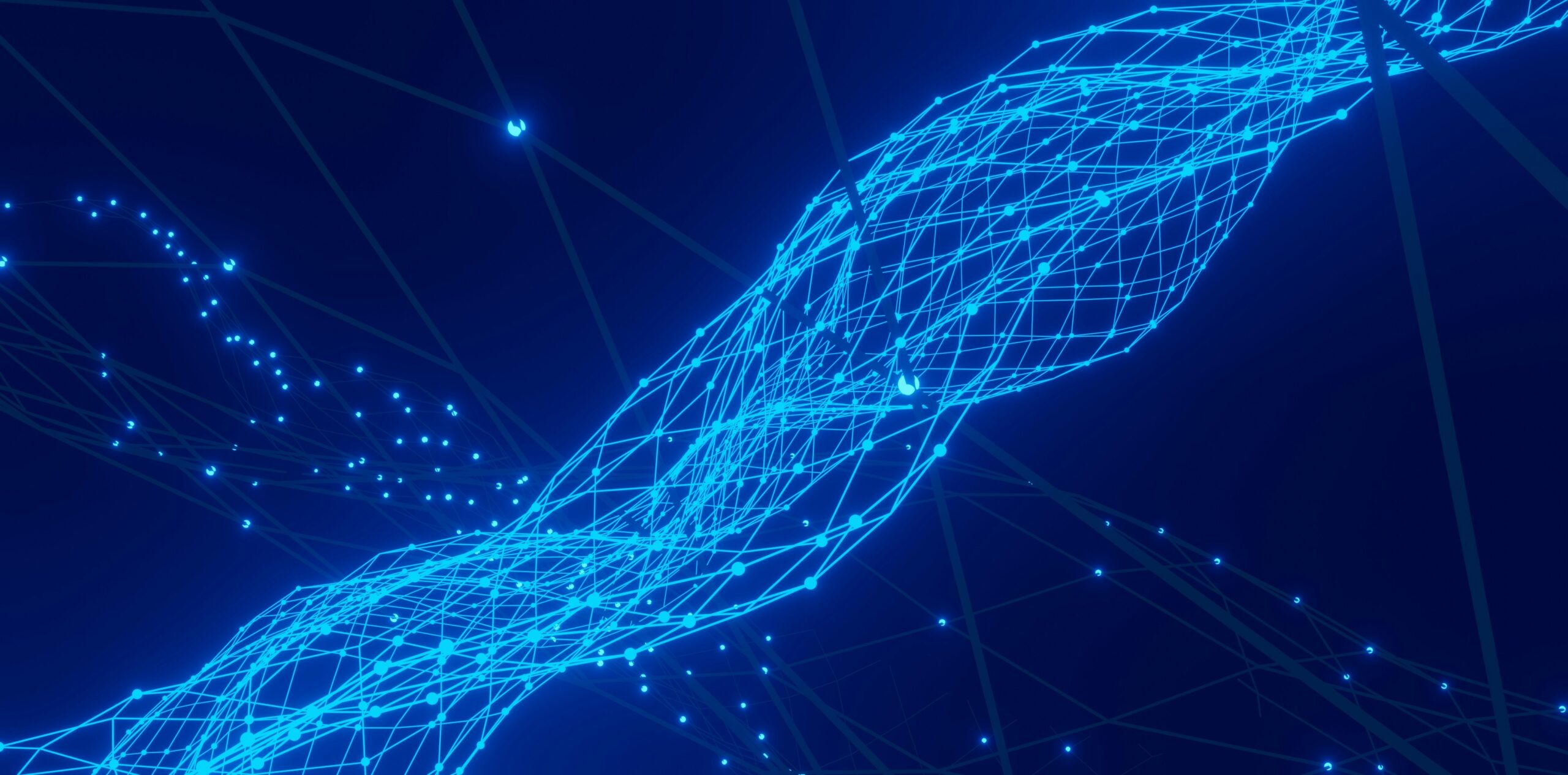
Skills Required for Data Scientists
Data science is a multifaceted discipline that necessitates a diverse set of skills and competencies to address complex problems effectively. At the forefront are programming languages, with Python and R emerging as the most widely utilized tools among data scientists. These languages offer robust libraries and frameworks that facilitate tasks such as data manipulation, statistical analysis, and machine learning. Proficiency in these languages allows data scientists to efficiently handle large datasets and execute sophisticated algorithms.
In addition to programming, a strong foundation in statistical analysis is imperative. Statistics provides the fundamental techniques for collecting, analyzing, and interpreting data, enabling data scientists to draw meaningful insights and make data-driven decisions. Knowledge of concepts such as regression analysis, hypothesis testing, and statistical significance is crucial for designing experiments and validating results.
Machine learning further enhances the capabilities of data scientists by enabling them to build predictive models and automate decision-making processes. Familiarity with various machine learning algorithms, such as supervised and unsupervised learning techniques, empowers professionals to develop solutions tailored to specific business challenges. Moreover, skills in implementing frameworks like TensorFlow and scikit-learn are increasingly sought after in the job market.
Data visualization tools are another essential component of a data scientist’s toolkit. Tools like Tableau, Power BI, and Matplotlib facilitate the effective visualization of complex data, making insights easier to communicate to stakeholders. The ability to craft compelling visual narratives around data not only aids in understanding but also enhances stakeholder engagement.
Lastly, soft skills should not be overlooked. Problem-solving abilities and excellent communication skills are paramount for data scientists. These competencies enable professionals to dissect business problems, collaborate with cross-functional teams, and present their findings in a manner that resonates with various audiences. As the field of data science continues to evolve, striking a balance between technical prowess and interpersonal skills will be vital for success.
Conclusion and Key Takeaways
Data science has emerged as a pivotal function in the decision-making process across various sectors, effectively reshaping how organizations approach challenges and opportunities. By harnessing vast amounts of data, professionals are equipped with the necessary insights to guide their choices, ultimately leading to more informed and strategic outcomes. Throughout this blog post, several key themes have been addressed, emphasizing how data science, through the rigorous analysis of data, can illuminate trends that might otherwise go unnoticed.
The importance of confiler systems in data science cannot be overstated. These systems facilitate efficient data management and analysis, enabling data scientists to perform complex computations seamlessly. As a result, organizations can optimize their operations and make evidence-based decisions. Furthermore, the integration of advanced analytics and machine learning techniques has propelled the field of data science forward, enabling predictive modeling and enhancing overall performance.
Another vital aspect discussed is the need for continuous learning within the data science community. As technology evolves at an unprecedented pace, so too do the tools and methodologies within the discipline. It is essential for professionals to stay informed and adapt to these changes, ensuring they remain relevant and capable of delivering actionable insights. Engaging in ongoing training, participating in professional networks, and following emerging trends are all ways practitioners can enhance their expertise.
In summary, the role of data science in contemporary decision-making extends beyond mere data analysis; it encapsulates a strategic approach to problem-solving that is highly valuable across various domains. By embracing the principles of data science and its various applications, organizations can harness the power of data and drive sustainable growth and innovation in an increasingly complex environment.
Read more blogs https://eepl.me/blogs/
For More Information and Updates, Connect With Us
- Name: Sumit Singh
- Phone Number: +91-9835131568
- Email ID: teamemancipation@gmail.com
- Our Platforms:
- Digilearn Cloud
- EEPL Test
- Live Emancipation
- Follow Us on Social Media:
- Instagram – EEPL Classroom
- Facebook – EEPL Classroom