Introduction to Machine Learning and AI
In today’s rapidly evolving technology-driven landscape, the significance of machine learning and artificial intelligence (AI) cannot be overstated. These disciplines play a crucial role in various applications, ranging from personalized recommendations to autonomous vehicles. At their core, machine learning refers to the process through which systems learn from data, allowing for the development of models that can make predictions or decisions without explicit programming. AI, on the other hand, encompasses a broader category of technologies that simulate human-like intelligence, enabling machines to perform tasks that typically require cognitive functions.
The historical roots of AI trace back to the mid-20th century when pioneers such as Alan Turing and John McCarthy proposed foundational theories for machines to mimic human thought processes. Turing’s concept of a “universal machine” laid the groundwork for future developments, while McCarthy’s coining of the term “artificial intelligence” in 1956 marked the inception of a field that continues to grow. Over the decades, significant milestones have occurred, such as the invention of neural networks in the 1980s and their resurgence due to increased computational power and vast amounts of data in the 2000s, marking a turning point in advancements in machine learning and artificial intelligence.
Today, the scope of machine learning has expanded to include techniques such as deep learning, reinforcement learning, and supervised and unsupervised learning approaches. These advancements empower machines to analyze and interpret complex data, driving innovations across various sectors like healthcare, finance, and transportation. As machine learning and AI continue to evolve, they are revolutionizing how we interact with technology, transforming industries, and presenting new challenges and opportunities. Understanding these domains is essential for grasping the future trajectory of digital innovation.
Key Milestones in AI Development
The evolution of artificial intelligence (AI) and machine learning has been marked by significant milestones that have shaped their development and integration across various sectors. The journey commenced in the mid-20th century, with Alan Turing’s groundbreaking work on computation and intelligence. In 1956, the Dartmouth Conference officially marked the birth of AI as a field of study, bringing together researchers who aimed to create machines capable of intelligent behavior.
Throughout the 1960s and 1970s, researchers developed early AI programs that demonstrated promise. Notable achievements during this period included the creation of ELIZA, an early natural language processing program that simulated conversation, and the development of perceptrons, laying the groundwork for neural networks. The subsequent winter of AI research in the 1980s, caused by a lack of funding and unmet expectations, highlighted the challenges and limitations of the technology at the time.
A renaissance in AI began in the late 1990s and early 2000s, fueled by substantial advancements in computing power and data availability. The introduction of support vector machines and decision trees revolutionized machine learning algorithms, enabling more sophisticated data processing and analysis. In 2012, a pivotal moment arrived with the breakthrough of deep learning, demonstrated through the success of neural networks in image recognition tasks during the ImageNet competition.
Another significant milestone occurred in 2016, when Google’s DeepMind developed AlphaGo, an AI program that defeated the world champion Go player. This landmark achievement showcased the capabilities of machine learning and reinforced the potential applications in complex problem-solving scenarios. The integration of AI in various domains, including healthcare, finance, and transportation, has continued to grow exponentially, with advancements in machine learning facilitating better predictions and enhanced decision-making processes.
As we observe the rapid pace of innovation today, the advancements in machine learning and artificial intelligence signal a future filled with endless possibilities. These milestones not only reflect past accomplishments but also set the stage for a new era in technology and intelligence.
Recent Innovations in Machine Learning Techniques
Machine learning has witnessed remarkable advancements that are driving innovations across multiple industries. Among these advancements, deep learning, reinforcement learning, and generative adversarial networks (GANs) stand out as transformative techniques that enable machines to learn and make decisions more efficiently than ever before.
Deep learning, a subset of machine learning, leverages neural networks with many layers to discover intricate patterns in vast amounts of data. This technique has proven to be particularly effective in image and speech recognition, powering applications in healthcare diagnostics and virtual assistants. For instance, deep learning algorithms can analyze medical images to identify early signs of diseases such as cancer, thereby enhancing patient outcomes.
Reinforcement learning, another innovative technique, involves training algorithms through rewards and penalties. In this scenario, an AI agent learns to make sequential decisions by interacting with its environment to maximize cumulative rewards. This method has shown its potential in robotics and autonomous systems, enabling machines to adapt their strategies in real time. Industries such as finance are also leveraging reinforcement learning for algorithmic trading, where models learn to optimize investment strategies based on market data.
Generative adversarial networks (GANs) represent a groundbreaking approach within the machine learning landscape. This technique involves two neural networks, a generator and a discriminator, that compete against each other, leading to the generation of new, realistic data. GANs have found applications in creative fields such as art and music, as well as in enhancing synthetic data generation for training other machine learning models. These innovations illustrate the vast potential of GANs to drive creativity and improve data diversity.
As these techniques continue to evolve, the impact of advancements in machine learning and artificial intelligence is profound, ushering in new solutions that enhance operational efficiency and create opportunities across various domains.
AI in Everyday Life: Applications and Use Cases
As advancements in machine learning and artificial intelligence continue to evolve, their practical applications permeate various aspects of daily life, significantly enhancing user experiences and driving efficiencies across multiple domains. One of the most visible applications is found in smart home devices. These gadgets, equipped with AI, allow users to manage and automate home functions such as lighting, heating, and security systems. For instance, smart thermostats learn the preferences of users over time, adjusting temperatures automatically to optimize comfort and energy savings.
Another noteworthy application is the proliferation of virtual assistants such as Amazon’s Alexa, Google Assistant, and Apple’s Siri. These AI-driven tools leverage natural language processing to understand user commands and execute tasks ranging from setting reminders to providing real-time information on weather and traffic. Such virtual assistants have become integral components of everyday routines, simplifying the management of tasks and providing instant access to information.
Advancements in machine learning also show a substantial impact within the realm of recommendation systems. Services like Netflix and Spotify utilize algorithms based on user behavior to analyze preferences, thereby generating tailored suggestions for movies, shows, or music. This not only enhances user engagement but also ensures that consumers discover content that aligns more closely with their tastes.
Moreover, industries such as healthcare have embraced AI technologies to refine patient diagnoses and treatment plans. Machine learning applications are increasingly employed to analyze medical records and predict patient outcomes, enabling practitioners to offer more personalized and effective care. With these advancements, the intersection of AI and daily life is unmistakable, showcasing the technology’s potential to transform routines and optimize efficiency in numerous sectors.
Ethics and Challenges in AI Development
The rapid advancements in machine learning and artificial intelligence have brought forth numerous ethical considerations that must be addressed to ensure responsible development and implementation. One of the primary challenges concerns the presence of bias in algorithms. Machine learning models are trained on data that often reflects societal biases, which can lead to skewed results and reinforce discrimination in critical areas such as hiring, law enforcement, and healthcare. As a result, it is imperative for developers to actively identify and mitigate biases within their datasets and models to foster fairness and inclusivity in AI applications.
Data privacy is another significant challenge in the realm of artificial intelligence. With the increasing reliance on vast amounts of personal data to train AI systems, concerns regarding data security and user consent have emerged. The potential for misuse or unauthorized access to sensitive information can jeopardize individual privacy rights and erode public trust in AI technologies. Establishing robust data governance frameworks and promoting transparency in how data is collected, stored, and utilized is crucial to maintaining consumer confidence and ensuring ethical compliance.
Moreover, the implications of AI on jobs and society cannot be overlooked. Automation driven by advancements in machine learning is expected to disrupt traditional job markets, rendering certain skill sets obsolete while simultaneously creating new opportunities. This transition necessitates careful consideration of workforce retraining and educational initiatives, as well as policies designed to address the economic disparities that may arise. Balancing technological innovation with its societal impact is vital to fostering sustainable growth in an AI-driven economy.
In conclusion, addressing the ethical considerations and challenges posed by advancements in machine learning and artificial intelligence is essential. By prioritizing fairness, data privacy, and the well-being of society, stakeholders can help ensure that AI technologies are developed responsibly and inclusively, paving the way for a more equitable future.
Future Trends and Predictions for AI and Machine Learning
The landscape of artificial intelligence (AI) and machine learning (ML) is on the brink of significant transformation, influenced by multiple emerging trends that promise to redefine numerous sectors. One of the most pivotal advancements in machine learning and artificial intelligence is the exploration of quantum computing. This technology holds the potential to exponentially increase processing power, allowing for more complex algorithms and faster data analysis. By harnessing quantum mechanics, businesses could leverage real-time insights and predictions that were previously unattainable, leading to enhanced decision-making processes across various industries.
Another important trend is the rise of AI-powered creativity, wherein algorithms and deep learning models are being employed to generate artistic works, music, and even literature. This fusion of technology and creativity is challenging traditional notions of authorship and originality, as AI tools provide a capability to mix, transform, or create unique content based on vast datasets. As these systems become more sophisticated, their role in creative fields may evolve, thereby influencing career trajectories for artists and content creators alike.
Additionally, further automation is anticipated to reshape the workforce significantly. As machine learning algorithms and AI continue to develop, routine tasks across various professions will likely be automated, raising questions about job displacement and the future of work. Many experts speculate that while certain jobs may become obsolete, new opportunities will arise to manage, supervise, and complement these automated systems. This shift could necessitate a re-evaluation of workforce skills, prompting educational institutions and training programs to adapt to the needs of a tech-enhanced labor market.
Overall, the convergence of these advancements will not only influence industries but also challenge societal norms and frameworks. As we move forward, it is crucial to engage in dialogues about the ethical implications and responsibilities associated with these technologies, ensuring that advancements in machine learning and artificial intelligence benefit society holistically.
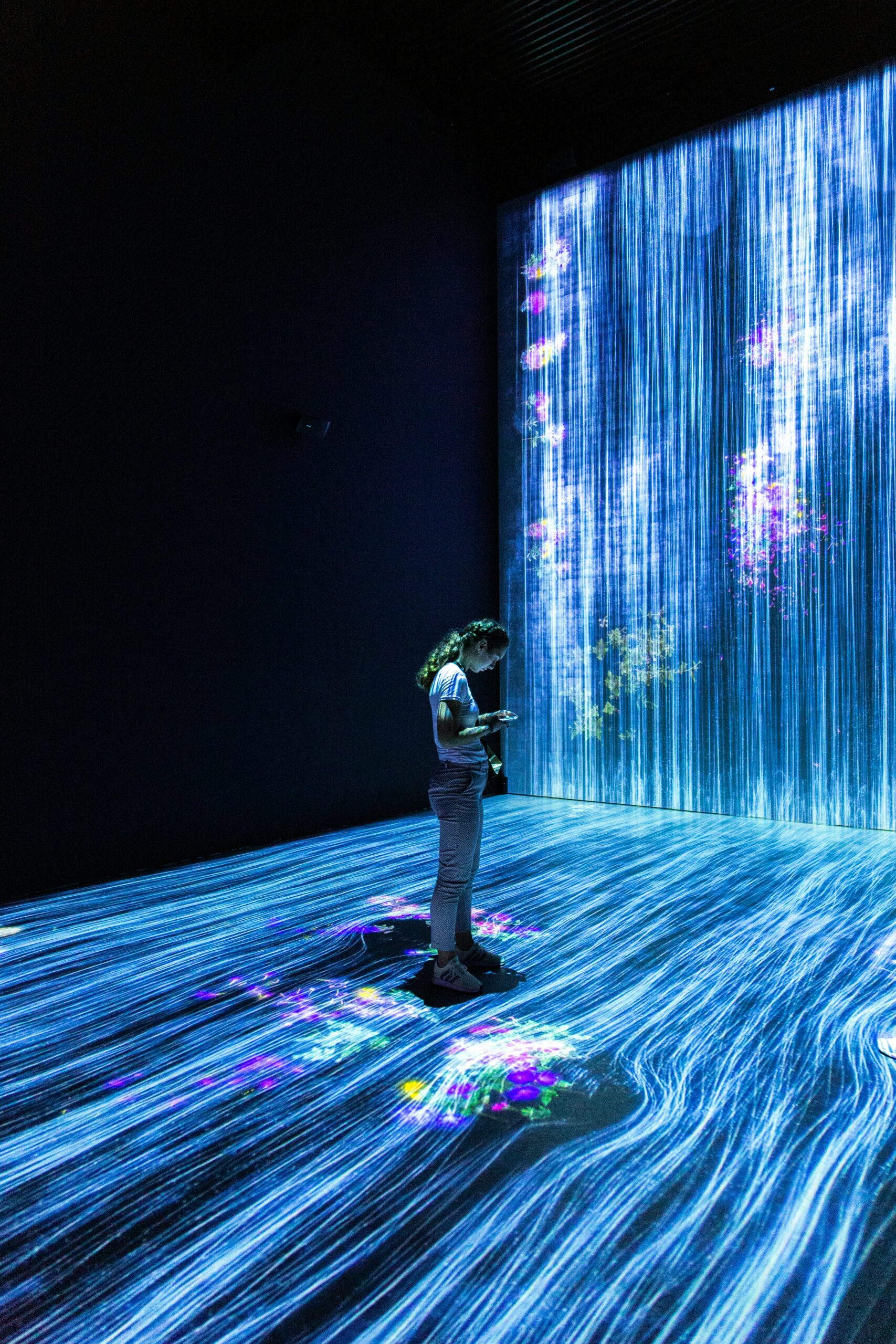
The Role of Big Data in AI Enhancements
The relationship between big data and advancements in machine learning and artificial intelligence is pivotal. Big data refers to the vast volumes of data generated every second, which include structured and unstructured information from myriad sources, such as social media, sensors, transaction records, and IoT devices. The sheer scale and diversity of this data provide a rich foundation for training machine learning models, enabling them to learn from patterns and make predictions with a heightened degree of accuracy.
As machine learning algorithms analyze large datasets, they refine their predictive capabilities. For instance, in applications such as natural language processing and image recognition, the exposure to extensive and varied data sources allows learning models to discern subtle nuances that smaller datasets may fail to reveal. Consequently, organizations leverage these advancements in artificial intelligence to deliver tailored experiences to users, thereby enhancing customer satisfaction and engagement.
However, the integration of big data within AI systems is not without its challenges. Data sourcing is a critical area that requires attention; acquiring high-quality, relevant datasets can be difficult. Moreover, organizations must navigate concerns regarding data privacy, storage, and management. The process of cleaning and preparing data for analysis is equally essential, as the effectiveness of machine learning models relies heavily on the accuracy and reliability of the input data. Without effective data management strategies, the anticipated benefits of machine learning may not be fully realized.
In conclusion, the synergy between big data and advancements in machine learning and artificial intelligence significantly enhances the capabilities of AI systems. By harnessing the power of vast datasets, organizations are better positioned to create innovative solutions that cater to user needs while addressing the complexities inherent in data management and privacy.
AI in Industry: Transformations Across Sectors
The integration of advancements in machine learning and artificial intelligence has led to transformative changes across various sectors, significantly enhancing operational efficiencies and decision-making processes. The healthcare industry stands out as one of the most impacted by these technologies. AI algorithms analyze vast amounts of medical data to diagnose diseases earlier, recommend personalized treatment plans, and assist in drug discovery. For instance, predictive analytics in patient care not only improves outcomes but also reduces costs associated with prolonged hospital stays.
In the automotive sector, machine learning has ushered in innovations such as autonomous vehicles, which leverage AI systems to enhance safety and navigation. These vehicles utilize sensors and real-time data to make informed driving decisions, minimizing risks of accidents. Furthermore, AI’s role in predictive maintenance allows manufacturers to foresee potential mechanical issues before they arise, ensuring operational continuity and safety.
The finance industry is also experiencing notable shifts due to machine learning and AI technologies. Financial institutions employ these advancements to analyze market trends, assess credit risks, and detect fraudulent transactions effectively. Automated processes enabled by AI improve customer service through chatbots and personalized financial advice, thereby streamlining operations and enhancing user experiences.
In manufacturing, AI-powered robotics are transforming production lines by optimizing processes, enhancing supply chain management, and executing complex tasks with precision. The implementation of machine learning algorithms enables manufacturers to adapt to market demands swiftly, thus fostering innovation and competitiveness. The continual evolution of these technologies presents opportunities for improvement in efficiency, cost reduction, and quality enhancement across sectors.
As various industries leverage advancements in machine learning and artificial intelligence, the potential for growth and innovation becomes increasingly apparent, demonstrating a profound impact on both operational and strategic levels.
Conclusion: Embracing the AI Revolution
The ongoing advancements in machine learning and artificial intelligence represent a profound shift in technology that is reshaping numerous aspects of daily life and various industries. As we have explored throughout this blog post, these innovations have the potential to enhance productivity, improve decision-making processes, and lead to unprecedented opportunities across sectors such as healthcare, finance, and education. The capabilities of AI systems to analyze vast datasets and derive actionable insights underscore their significance in contemporary society.
However, the rapid integration of artificial intelligence into our lives does not come without challenges. Ethical considerations are paramount as we delve deeper into how machine learning applies to sensitive areas like personal data, privacy, and systemic biases. It is crucial for policymakers, technologists, and society at large to address these implications collaboratively. By setting appropriate regulations and ensuring responsible usage of AI technologies, we can mitigate risks and foster an environment where advancements in machine learning contribute positively to society.
Moreover, embracing this AI revolution involves continuous learning and adaptation. Individuals and organizations must stay informed about the latest developments in artificial intelligence and machine learning to remain competitive and conscientious. This requires an investment not only in technological infrastructure but also in educational initiatives aimed at equipping the workforce with the necessary skills to thrive in an AI-driven world.
In conclusion, the advancements in machine learning and artificial intelligence bring about both tremendous opportunity and significant responsibility. By recognizing the importance of ethical considerations and encouraging widespread collaboration, we can harness these technologies effectively and ensure they serve the greater good. The future of AI is not predetermined; it is a collective endeavor that requires society’s active participation to shape a better tomorrow.
- Name: Sumit Singh
- Phone Number: +91-9835131568
- Email ID: teamemancipation@gmail.com
- Our Platforms:
- Digilearn Cloud
- EEPL Test
- Live Emancipation
- Follow Us on Social Media:
- Instagram – EEPL Classroom
- Facebook – EEPL Classroom